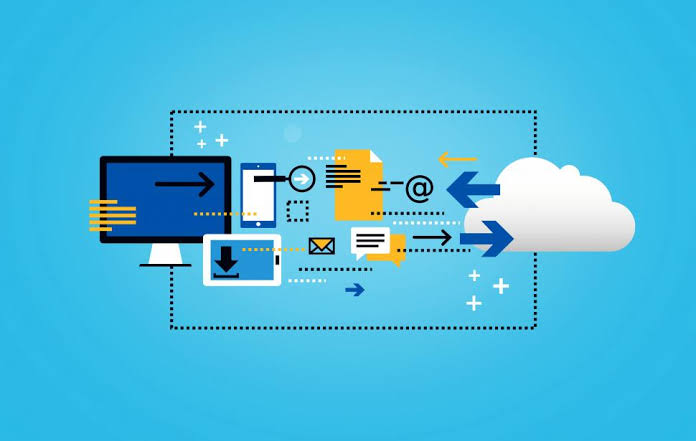
BIG DATA AND CYBER WORLD
– By MEGHA MALHOTRA
What is Big Data?
Big data is a blend of structured, semi structured and unstructured data gathered by board structures in organisations, that can be dug for information and utilized in machine learning projects and AI ventures, predictive modeling and other advanced analytics applications.
Frameworks that process and store big data have become a typical component of data management architectures in organizations. Big data is often characterized by the 3Vs: the large volume of data in many environments, the wide variety of data types stored in big data systems and the velocity at which the data is generated, collected and processed. These attributes were first recognised by Doug Laney, then an analyst at Meta Group Inc., in 2001. More recently, several other Vs have been added to different descriptions of big data, including veracity, value and variability.
Although big data doesn’t equate to any specific volume of data, big data deployments often involve terabytes (TB), petabytes (PB) and even exabytes (EB) of data captured over time.
Deciphering the Vs of Big Data
1. Volume is the most commonly cited characteristic of big data. A big data environment doesn’t have to contain a large amount of data, but most do because of the nature of the data being gathered and stored in them. Clickstreams, system logs and stream processing systems are among the sources that typically produce massive volumes of big data on an ongoing basis.
2. Big data also incorporates a wide variety of data types, including the following:
• structured data in databases and data warehouses based on Structured Query Language (SQL);
• unstructured data, such as text and document files held in Hadoop clusters or NoSQL database systems; and
• semi structured data, such as web server logs or streaming data from sensors.
This entirety of data types can be stored together in a data lake, which typically is based on Hadoop or a cloud object storage service. Moreover, big data applications often includes multiple data sources that may not otherwise be integrated. For example, a big data analytics project may attempt to gauge a product’s success and future sales by correlating past sales data, return data and online buyer review data for that product.
3. Velocity refers to the speed at which big data is generated and must be processed, analysed and broken down. In many cases, sets of big data are updated on a real- or near-real-time that is to say, genuine basis, instead of the daily, weekly or monthly updates made in many traditional data warehouses. Big data analytics applications ingest, correlate and ingests the incoming data and then render an answer or result based on an overarching query.
Managing data velocity is additionally significant as big data analysis expands into fields like machine learning and artificial intelligence (AI), where analytical processes automatically find patterns in the collected data and use them to generate insights.
4. Looking past the original 3Vs, data veracity refers to the degree of certainty in data sets. Uncertain raw data collected from multiple sources — such as social media platforms and webpages — can cause serious data quality issues that may be hard to pinpoint..
Bad data leads to inaccurate analysis and may undermine or subvert the business since it can cause executives to doubt data as a whole. The amount of uncertain data in an organization must be accounted for before it is used in big data analytics applications. IT and analytics teams also need to ensure that they have enough accurate data available to produce valid results.
5. Some data researchers also add value to the list of characteristics of big data. As explained above, not all data collected has real business esteem, and the use of inaccurate off base data can weaken the insights provided by analytics applications. It’s critical that organizations employ practices such as data cleansing and confirm that data relates to relevant business issues before they use it in a big data analytics project.
6. Variability likewise often applies to sets of big data, which are less consistent than conventional and traditional data and may have numerous implications or be formatted in different ways from one data source to another — factors that further complicate efforts to process and analyze the data. Some people ascribe even more Vs to big data; data scientists and consultants have created various lists with between seven and 10 Vs.
Big Data : A Saviour
“Big data powers the cybersecurity world. Modern cybersecurity solutions are mostly driven by big data.”
Some say Big Data is a threat; others proclaim it a Guardian angel. Big Data can store large amounts of data and help analysts examine, observe, and detect abnormalities within a network. That makes Big Data analytics an appealing idea to help escape cybercrimes.
The security-related data accessible from Big Data reduces the time required to detect and resolve an issue, permitting cyber analysts to predict and avoid the potential outcomes of intrusion and invasion. According to a CSO Online report, 84% of business use Big Data to help block or obstruct these attacks. They also reported a decent decline in security breaches after introducing Big Data analytics into their operations.
Insights from Big Data analytics tools can be used to detect cybersecurity threats, including malware/ransomware attacks, compromised and weak devices, and malicious insider programs. This is where Big Data analytics looks most promising in improving cybersecurity. The respondents in the CSO Online survey acknowledge that they can’t use the power of Big Data analytics to its full potential for several reasons, such as the overwhelming volume of data; lack of the right tools, systems, and experts; and obsolete data. Big Data doesn’t provide rock-solid security due to poor mining and the absence of experts who know how to use analytics trends to fix gaps.
Threat visualization
Big Data analytics programs can help you predict the class and intensity of cybersecurity threats. You can weigh the complexity of a possible attack by evaluating data sources and patterns. These tools also permit you to use present and historical data to get statistical understandings of which trends are acceptable and which are not.
There are three principle challenges that organizations are running into with big data :
• Protecting sensitive and personal information
• Data rights and ownership
• Not having the talent (i.e. data scientists) to analyze the data
While meeting the fundamental challenge of safeguarding data may sound simple enough, when you look at the scale of data that needs to be processed and analyzed in order to prevent cyber attacks, the challenge becomes a little more daunting.
Traditionally, the technologies or innovations and security tools that have been used to dug data and prevent cyber attacks have been more reactive than proactive and have likewise created a large number of false positives, creating inefficiencies and distracting from actual threats. What’s more these conventional tools do not have the bandwidth required to deal with the large volumes of information.
Big Data as an Opportunity
In comparison, big data analytics enable cyber security professionals the ability to analyze data from various sources and data types and then respond in real time. Big data analytics is not only able to accumulate information from a vast universe but it is also able to connect the dots between data, making correlations and connections that may have otherwise been missed. This increases efficiencies for cyber crime professionals and casts a wider more reliable net when it comes to thwarting cyber attacks.
If businesses can figure out how to use modern technologies and inventions to safeguard personal and sensitive data, then the opportunities that big data present are great. The two biggest benefits big data offers companies today are:
• Business intelligence through access to vast data/customer analytics that can be used to enhance and optimize sales and marketing strategies
• Fraud detection and a SIEM systems replacement
Intelligent risk management : To improve your cybersecurity efforts, your tools must be backed by intelligent risk-management insights that Big Data experts can easily interpret. The key purpose of using these automation tools should be to make the data accessible to analysts more easily and quickly. This approach will allow experts to source, categorize, and handle security threats without delay.
Predictive models : Intelligent Big Data analytics enables experts to build a predictive model that can issue an alert as soon as it sees an entry point for a cybersecurity attack. Machine learning and artificial intelligence can play a major role in developing such a mechanism. Analytics-based solutions enable you to predict and gear up for possible events in your process.
Securing systems with penetration testing
Infrastructure penetration testing will give you insight for your business database and process and help keep hackers at bay. Penetration testing is a simulated malware attack against your computer framework and network to check for exploitable vulnerabilities. It is like a mock-drill exercise to check the capabilities of your process and existing analytics solutions. Penetration testing has become an essential step to protect IT infrastructure and business data.
Penetration testing involves five stages:
1. Planning and reconnaissance
2. Scanning
3. Gaining access
4. Maintaining access
5. Analysis and Web application firewall (WAF) configuration
The outcomes shown by a penetration test exercise can be used to enhance the fortification of a process by improving WAF security policies.
Once you have configured your policies and strengthened your process, you can do a new penetration test to gauge the adequacy of your preventive measures.
Sometimes vulnerabilities in an infrastructure are right in front of the analysts and property owners and still manage to go unnoticed. Operating systems, services and application flaws, improper configurations, and risky end-user behavior are some of the most common places where cybersecurity vulnerabilities exist.
Strengthening Big Data Security
When cyber criminals target big data sets, the reward is often well worth the effort needed to penetrate security layers, which is why big data presents such a great opportunity not only for businesses but for cyber criminals. They have a lot more to gain when they go after such a large data set. Subsequently, companies have a lot more to lose should they face a cyber attack without the proper security measures in place.
In order to increase the security around big data, your business may consider:
• Collaborating with other industry peers to create industry standards, head off government regulations, and to share best practices
• Attribute based encryption to protect sensitive information shared by third parties
• Secure open source software such as Hadoop
• Maintain and monitor audit logs across all facets of the business
Overall, big data presents gigantic opportunities for businesses that go beyond just enhanced business intelligence. Big data offers the ability to increase cyber security itself. Yet, in order to benefit from the many opportunities big data presents, companies must shoulder the responsibility and risk of protecting that data.
Bottom line
Big Data analytics solutions, backed by machine learning and artificial intelligence, give hope that businesses and processes can be kept secure in the face of a cybersecurity breach and hacking. Employing the power of Big Data, we can improve our data-management techniques and cyber threat-detection mechanisms.
Monitoring and improving your approach can bulletproof your business. Periodic penetration tests can help ensure that your analytics program is working perfectly and efficiently
There are reports that a large amount of data is going online for sale and most of it consist of Indian origin. If you know how to access darkweb you can access that information in around 70 dollars. This is a threat because these reports show that how easily they have got access to the information. We need a protocol for countering such threats , because an insecure data is no less than a full fledged terrorist attack.
Unlike the strict EU laws, in India we are far away from making the large brands like facebook, google to handle the data of our population very carefully and not to sell it to advertisers! Cheap smartphone brands which provide budget phones to almost 70% of our country, are able to cut their costs by making huge profits on selling user data!
We need much more effective laws and for that, we need people with better understanding of these threats, to suggest the ministers about the same.
Great Article
Well done Mam. Great research and study also perfectly delivered.
I would like to praise the author for writing this great and knowledgeable data over this topic. The information was effective and informative.Nice work